The NIMH Director’s Innovation Speaker Series
The National Institute of Mental Health (NIMH) is pleased to invite you to attend a series of lectures dedicated to innovation, invention, and scientific discovery. NIMH established the Director’s Innovation Speaker Series to encourage broad, interdisciplinary thinking in the development of scientific initiatives and programs, and to press for theoretical leaps in science over the continuation of incremental thought. Innovation speakers are encouraged to describe their work from the perspective of breaking through existing boundaries and developing successful new ideas, as well as working outside their primary area of expertise in ways that have pushed their fields forward. We encourage discussions of the meaning of innovation, creativity, breakthroughs, and paradigm-shifting.
Submit general questions to the NIMH Director’s Innovation Speaker Series mailbox.
Speaker Schedule 2024-2025
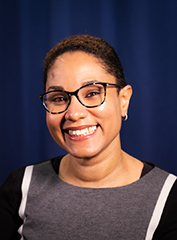
Arielle H. Sheftall, Ph.D.
Talk Title: Director's Innovation Speaker Series: Youth Suicidal Behaviors: Where Do We Go From Here
Date: December 16, 2024, 2:00–3:00 p.m. ET • Hybrid
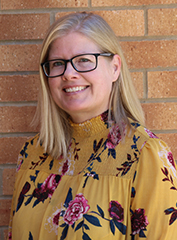
Megan Moreno, M.D., M.S.Ed., M.P.H. and Jenny Radesky, M.D.
Talk Title: Director’s Innovation Speaker Series: Youth-Centered Approaches to Media Research
Date: November 13, 2024, 2:00–3:00 p.m. ET • Hybrid
Speaker Schedule 2023-2024
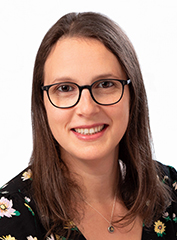
Anna Wexler, Ph.D.
Date: June 25, 2024, 2:00–3:00 p.m. ET • Hybrid
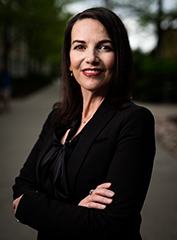
Julianne Holt-Lunstad, Ph.D.
Talk Title: Director’s Innovation Speaker Series: Social Connection: An eye to the past, present, and future
Date: October 26, 2023, 2:00-3:00 PM ET • Hybrid